
Runzi Wang
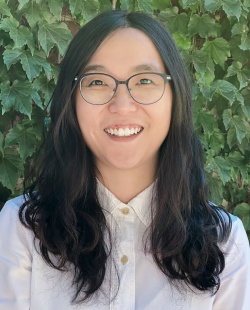
About
Runzi Wang, PhD, is a transdisciplinary researcher who studies change in natural and urban environments across space and over time, with the objective to drive positive change with ecological planning and design strategies. Combining technologies such as big data, machine learning, remote sensing, and spatial statistics, her primary research explores how land cover change and urban development pattern influence stream water quality and stormwater quality at the watershed basis, together with a variety of environmental, climatic and sociocultural factors. By enhancing the interpretability of machine learning in its application to landscape architecture, the most innovative part of her research is to uncover the nonlinear relationships between environmental, technological and sociocultural dimensions of landscape systems.
The focus areas of Runzi’s research include 1) Neighborhood-scale green infrastructure design optimization. 2) Regional-scale water quality management. 3) Continental-scale landscape change monitoring and projection. Runzi is also a landscape designer engaged in evidence-based landscape and urban design projects, with the focus on stormwater management. She integrates research with the teaching topics of ecological design studio, landscape analysis, and site engineering.
Publications
Guan, J., Wang, R*., Van Berkel, D., & Liang, Z. (2023). How spatial patterns affect urban green space equity at different equity levels: A Bayesian quantile regression approach. Landscape and Urban Planning, 233, 104709.
Wang, R., Ma, Y., Zhao, G.*, Zhou, Y., Shehab, I., & Burton, A. (2023). Investigating water quality sensitivity to climate variability and its influencing factors in four Lake Erie watersheds. Journal of Environmental Management, 325, 116449.
Goodspeed, R., Wang, R., Lizundia, C., Du, L., & Jaipuria, S. (2022). Incorporating water quality into land use scenario analysis with random forest models. Environment and Planning B: Urban Analytics and City Science, 23998083221138842.
Guzman, C. B., Wang, R.*, Muellerklein, O., Smith, M., & Eger, C. G. (2022). Comparing stormwater quality and watershed typologies across the United States: A machine learning approach. Water Research, 216, 118283.
Wang, R.*, Kim, J. H., & Li, M. H. (2021). Predicting stream water quality under different urban development pattern scenarios with an interpretable machine learning approach. Science of The Total Environment, 761, 144057.
Song, Y., Wang, R.*, Fernandez, J., & Li, D. (2021) Investigating sense of place of the Las Vegas Strip using online reviews and machine learning approaches. Landscape and Urban Planning, 205, 103956.
Wang, R.*, Zhang, X., & Li, M.-H. (2019). Predicting bioretention pollutant removal efficiency with design features: A data-driven approach. Journal of Environmental Management, 242, 403–414.
2023, PI, “Investigating the role of forest characteristics in mitigating climate change effects on water quality and fish community composition”. $138,000, USDA NIFA McIntire-Stennis. Collaborate with Karen Alof and Bill Curie
2022, PI, IRISE: “Intersecting Research Ideas towards Sustainable Environments-- Improving Built Environment Outcomes in the Face of Increasing Chemical and Pathogen Exposures”. $50,000, Bold Challenges Team Formation. Collaborate with Simone Charles (U-M public health) and Allen Burton
2022-2023, PI, “Investigating stream water contamination and prioritizing green stormwater infrastructure in the River Rouge Watershed from a socioecological perspective.” $5,000, Funded by Detroit Urban Research Center. Co-PI: Matthew Bertrand (Friends of the Rouge)
2022-2023, Co-PI, “Developing an Interdisciplinary Approach to Studying Urban Stream Water Quality.” $75,000, Funded by School for Environment and Sustainability. PI: Sara Hughes (U-M, SEAS), Co-PI: Seth Guikema (U-M, CoE)
2021-2022, PI, “Data science approach towards a socio-ecological framework for the investigation of continental urban stream water quality pattern.” $70,000, Funded by Michigan Institute for Data Science and Artificial Intelligence. Co-PI: Yang Chen (U-M, Statistics), Bill Currie (U-M, SEAS)
2021-2022, PI, “Urban water quality management towards a sustainable framework— the investigation of fine scale urban form effects on stream water quality”. $10,000, Funded by Graham Sustainability Institute Catalyst Grant Program. Co-PI: Robert Goodspeed (U-M, Taubman), Branko Kerkez (U-M, CoE), Joshua Newell (U-M SEAS), Yang Chen (U-M Statistics)
Michigan State University (PhD, Environmental Design) 2018-2020
Texas A&M University (Urban and Regional Sciences) 2014-2017
Peking University (MS, Landscape Architecture) 2011-2013
Shandong University (BE, Architecture) 2006-2011